Researchers at MIT have made a remarkable progress in equipping artificial intelligence (AI) with a form of peripheral vision similar to that of humans. The ability to detect objects outside the direct line of sight, albeit with less detail than that of humans, is a fundamental aspect of human vision. Now, scientists aim to replicate this capability in AI systems to enhance their understanding of visual scenes and potentially improve safety measures in various applications.
For this, the MIT team, led by Anne Harrington, developed an innovative image dataset to help AI models simulate peripheral vision. Through training with this dataset, they observed improvements in object detection capabilities.
A surprising finding was humans’ ability to detect objects in their periphery, outperforming AI models. Despite various attempts to enhance AI vision, including training from scratch and fine-tuning pre-existing models, machines consistently lagged behind human capabilities, particularly in detecting objects in the far periphery.
“There is something fundamental going on here. We tested so many different models, and even when we train them, they get a little bit better but they are not quite like humans. So, the question is: What is missing in these models?” says Vasha DuTell, a co-author of a paper.
This research points towards the complexities of human vision and the challenges in replicating it artificially. Harrington and her team plan to further research on these disparities, aiming to develop AI models that accurately predict human performance in peripheral vision tasks.
“Modeling peripheral vision, if we can really capture the essence of what is represented in the periphery, can help us understand the features in a visual scene that make our eyes move to collect more information,” DuTell explains.
Moreover, this work emphasizes the importance of interdisciplinary collaboration between neuroscience and AI research. By drawing insights from human vision mechanisms, scientists can refine AI systems to better mimic human perception, leading to more reliable applications.
The team initially utilized a method called the texture tiling model, commonly employed in studying human peripheral vision, to enhance accuracy. They then tailored this model to offer greater flexibility in altering images without requiring prior knowledge of the observer’s focal point.
“That let us faithfully model peripheral vision the same way it is being done in human vision research,” says Harrington.
In brief, this research marks a significant progress in bridging the gap between human and artificial vision. Such advancements could revolutionize safety systems, particularly in contexts like driver assistance technology, where detecting hazards in the periphery is crucial.
What’s even more interesting here is that, while neural network models have advanced, they still cannot match human performance in this area. This underscores the need for more AI research to gain insights from human vision neuroscience. The database of images provided by the authors will greatly aid in this future research.
This work, set to be presented at the International Conference on Learning Representations, is supported, in part, by the Toyota Research Institute and the MIT CSAIL METEOR Fellowship.
Have you ever tried this AI with a wonderful sense of humor? Visit this online platform where you can transform text into expressive speaking avatars!
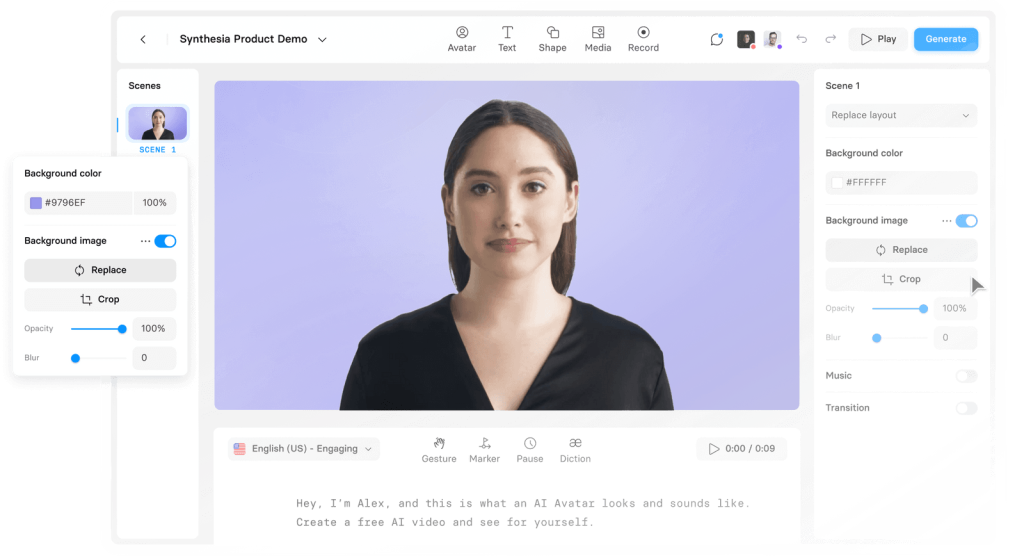
- AI-Powered PCs: Overhyped Trend or Emerging Reality? - August 21, 2024
- Princeton’s AI revolutionizes fusion reactor performance - August 7, 2024
- Large language models could revolutionize finance sector within two years - March 27, 2024