After being trained on real astronomical observations, artificial intelligence (AI) algorithms now outperform astronomers in sifting through massive amounts of data to find new exploding stars, identify new types of galaxies, and detect the mergers of massive stars, accelerating the rate of discovery in the world’s oldest science.
Astronomers say AI can reveal something deeper like unsuspected connections hidden in the complex mathematics arising from general particular, how that theory is applied to finding new planets around other stars.
What is an AI algorithm?
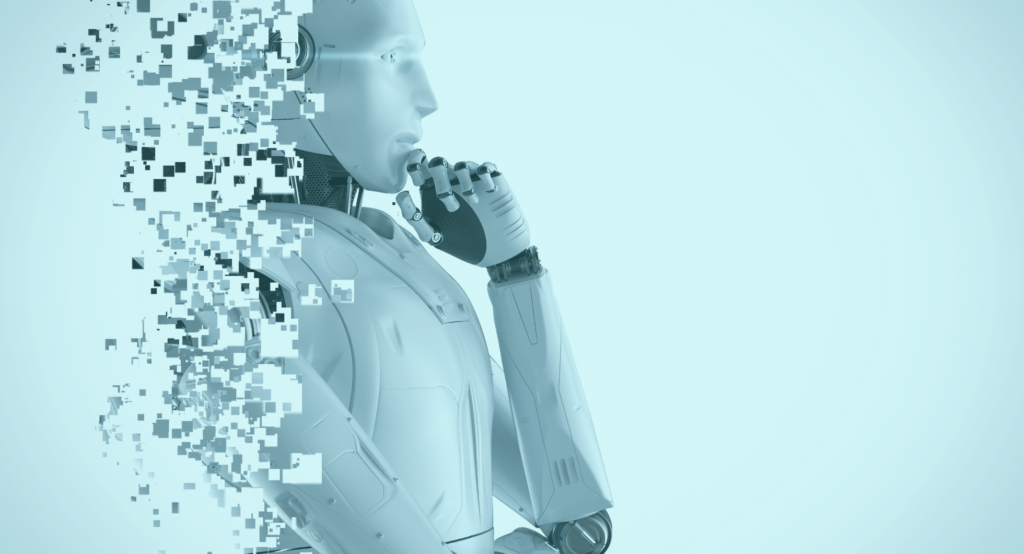
An AI algorithm is a set of mathematical instructions based upon some pattern or problem; and that the person creating the algorithm wants to solve. To do this, it uses known data sets (e.g., many different training sets) to solve similar problems by searching for patterns in the data. When given a new problem, it searches through its training data sets; and it utilizes whatever solution it finds to compare its new unknown results.
It then uses this comparison to alter its approach in an attempt to improve its solution for all future comparisons that use similar approaches. In practice, this means it will adjust itself based on the historical performance of the approach; it also makes future comparisons more accurate than past ones.
New finding: AI reveals unsuspected math underlying search for exoplanets
In revealing unsuspected math underlying the search for exoplanets, AI can offer insights into observations of massive stars and their remnants, including black holes and neutron stars. Moreover, AI can be used to develop more efficient ways of discovering new exoplanets; and possibly, we can use it for discovering life outside our solar system.
In a paper published this week in the journal Nature Astronomy, the researchers have described how an AI algorithm developed to more quickly detect exoplanets when such planetary systems pass in front of a background star and briefly brighten it. It’s a process called gravitational microlensing. It revealed that the decades-old theories now used to explain these observations are woefully incomplete.
Albert Einstein himself 1936 used his new theory of general relativity to show how the light from a distant star can be bent by the gravity of a foreground star, not only brightening it as seen from Earth but often splitting it into several points of light or distorting it into a ring, now called an Einstein ring. Researchers say this is similar to the way a hand lens can focus and intensify light from the sun.
Major degeneracies and their unification
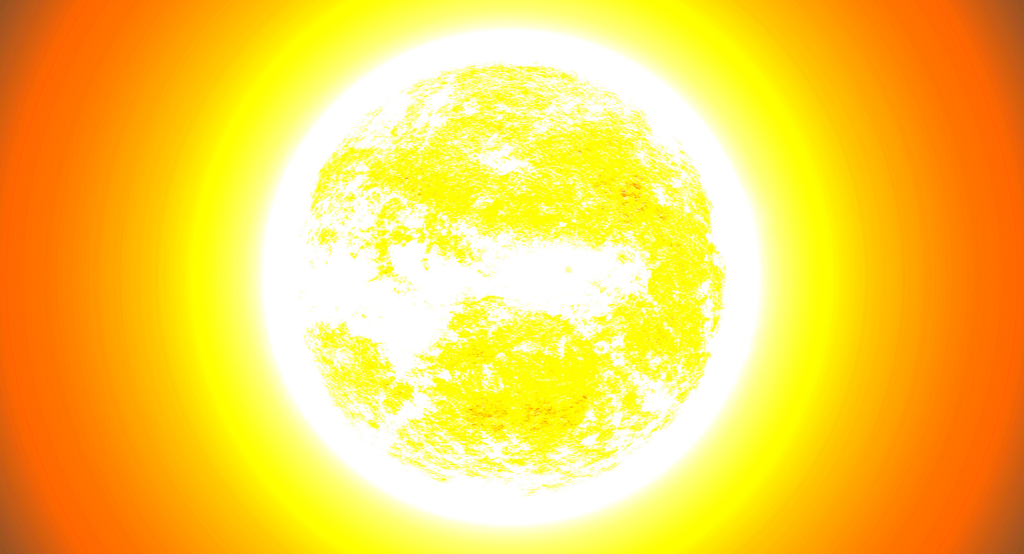
Degeneracies are the collective phenomenon arising from the massive stars in the center of the image. They cause light rays to split into many images that are seen as a ring.
An event related to gravitational microlensing was observed in 1987. At that time, two massive stars collided and caused their respective Einstein rings to merge into a single distorted ring. These events are called ‘symbiotic’ gravitational microlensing events. It’s because researchers say, they unite (or “symbolize”) several degenerate objects into a single one.
These degeneracies have no theory underlying them, so explaining them was thought impossible until now. AI has revealed hidden structures in this complex math. And that may show how it works for both general relativity and quantum mechanics.
When the foreground object is a star with a planet, the brightening over time — the light curve — is more complicated. What’s more, there are often multiple planetary orbits that can explain a given light curve equally well — so-called degeneracies. That’s where humans simplified the math and missed the bigger picture.
Also read: How can we make sure that AI does what it is supposed to do?
But the AI algorithm pointed to a mathematical way to unify the two major kinds of degeneracy in interpreting what telescopes detect during microlensing, showing that the two “theories” are really special cases of a broader theory that the researchers admit is likely still incomplete.
Now, professional astronomers say that a machine learning inference algorithm, which was previously developed, led us to discover something new and fundamental about the equations that govern the general relativistic effect of light- bending by two massive bodies.
Authors of the paper claim that this is kind of a milestone in AI and machine learning. They say Keming’s machine learning algorithm uncovered that degeneracy that had been missed by experts in the field toiling with data for decades. And this was suggestive of how research is going to go in the future when it is aided by machine learning, which is exciting.
Discovery of exoplanets to date and future plans
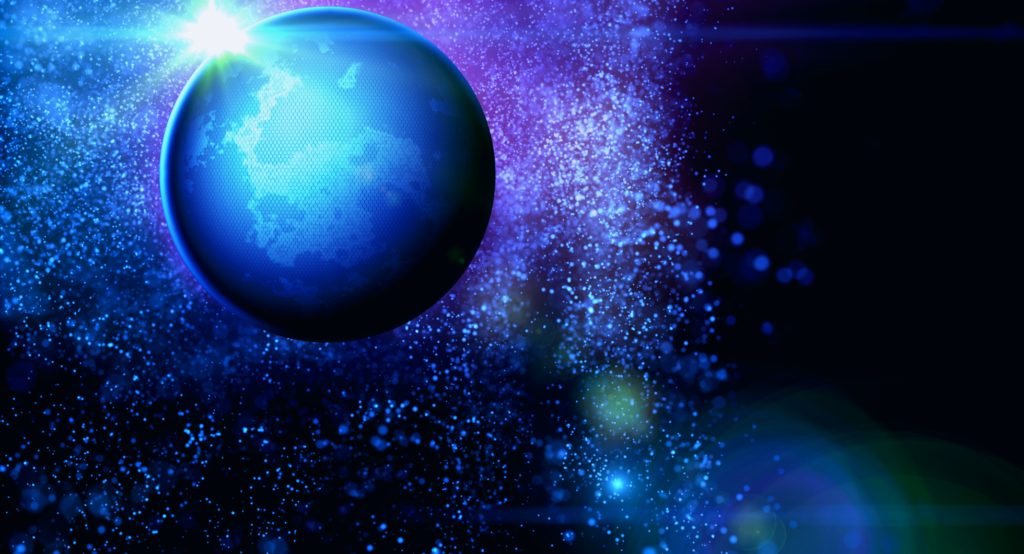
To date, more than 5,000 exoplanets, or extrasolar planets, have been discovered around stars in the Milky Way. However, few of them have been seen through a telescope and they are too dim. Most of them have been detected because they create a Doppler wobble in the motions of their host stars. Or because they slightly dim the light from the host star when they cross in front of it; transits that were the focus of NASA’s Kepler mission. And only a few more than 100 have been discovered by a third technique, microlensing.
NASA’s Nancy Grace Roman Space Telescope is scheduled to launch by 2027. It has the main goal to discover thousands more exoplanets via microlensing. NASA states that the technique has an advantage over the Doppler and transit techniques. It’s in that it can detect lower-mass planets, including those the size of Earth, that is far from their stars, at a distance equivalent to that of Jupiter or Saturn in our solar system.
Astronomers’ previous attempts to develop an AI algorithm
The team of Bloom, Zhang, and their colleagues set out two years ago to develop an AI algorithm to analyze microlensing data faster to determine the stellar and planetary masses of these planetary systems and the distances the planets are orbiting from their stars. They say that such an algorithm would speed the analysis of the likely hundreds of thousands of events the Roman telescope will detect to find the 1% or fewer that are caused by exoplanetary systems.
But one problem astronomers encounter is that the observed signal can be ambiguous. When a lone foreground star passes in front of a background star, the brightness of the background stars rises smoothly to a peak. And then it drops symmetrically to its original brightness. It’s easy to understand mathematically and observationally.
But if the foreground star has a planet, the planet creates a separate brightness peak within the peak caused by the star. While trying to reconstruct the orbital configuration of the exoplanet that produced the signal, general relativity often allows two or more so-called degenerate solutions; all of which can explain the observations.
View of astronomers on the issue
Scott Gaudi, the co-author of the paper, a professor of astronomy at The Ohio State University and one of the pioneers of using gravitational microlensing to discover exoplanets, said that astronomers have generally dealt with these degeneracies in simplistic and artificially distinct ways to date. If the distant starlight passes close to the star, we could interpret the observations either as a wide or a close orbit for the planet — ambiguity astronomers can often resolve with other data.
According to Gaudi, the second type of degeneracy occurs when the background starlight passes close to the planet. In this case, the two different solutions for the planetary orbit are generally only slightly different.
The researchers said that these two simplifications of two-body gravitational microlensing are usually sufficient to determine the true masses and orbital distances.
What’s in the new paper based on general relativity?
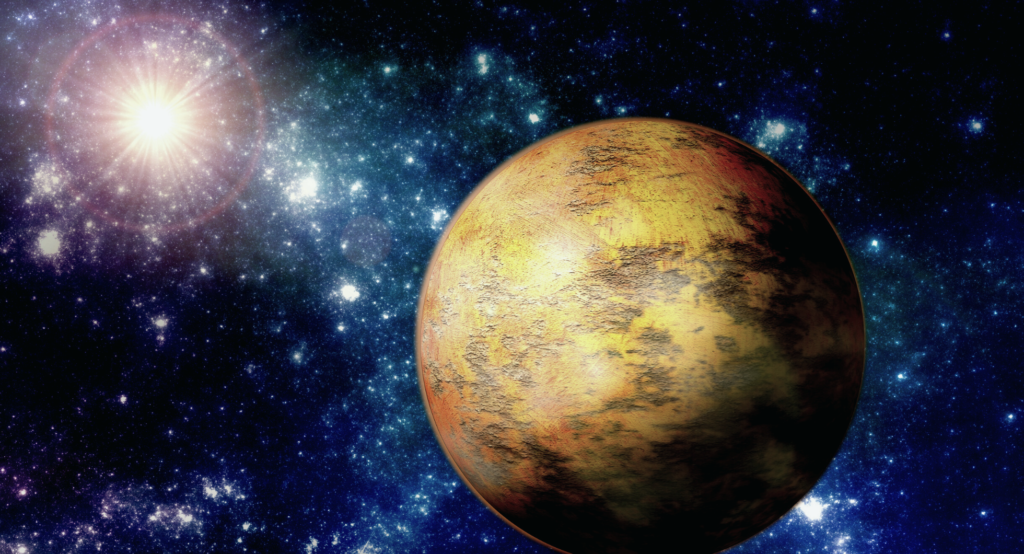
Zhang and Gaudi have submitted a new paper that rigorously describes the new mathematics based on general relativity. And it explores the theory in microlensing situations where more than one exoplanet orbits a star.
The new theory technically interprets microlensing observations with more ambiguity. It’s because there are more degenerate solutions to describe the observations. However, the theory also clearly demonstrates that observing the same microlensing event from two perspectives; it’s from Earth and the orbit of the Roman Space Telescope, for example. It will make it easier to settle on the correct orbits and masses. Gaudi also clarified that that is what astronomers currently have planned to do.
Likewise, Bloom said that the AI suggested a way to look at the lens equation in a new light and uncover something really deep about its mathematics of it.
So, for now, AI researchers, astronomers, and cosmologists are confident that machine learning is useful in understanding microlensing. They say that the new method has led to the discovery of a new mathematical property of general relativity. It could be useful for finding exoplanets with NASA’s Roman Space Telescope.
The team also says they may use their method to solve other issues in astrophysics. They have already found an interesting application in a separate field of stellar physics. There, researchers are trying to determine the average temperature and density of stars more accurately than they can now. These quantities are important for understanding how stars evolve over cosmic time and how they explode as supernovas.
- AI-Powered PCs: Overhyped Trend or Emerging Reality? - August 21, 2024
- Princeton’s AI revolutionizes fusion reactor performance - August 7, 2024
- Large language models could revolutionize finance sector within two years - March 27, 2024