Tomography-Derived Reconstructive Generative Network (tomoDRGN), the latest innovation in the field of molecular biology, is revolutionizing scientists’ ability to visualize proteins’ shape changes using advanced computational algorithms. MIT graduate student Barrett Powell and his colleagues have developed this new approach to understanding the structural dynamics of proteins within their native cellular environment.
Nowadays, cryogenic electron tomography (cryo-ET) has emerged as a favored technique for for studying proteins in their natural setting. By taking pictures of frozen cells from various angles, scientists can get a 3D view of protein structures. This is pretty cool because it lets researchers see exactly how and where proteins team up with each other, giving insights into their interactions within the cell.
With the current imaging technology for proteins in their natural setting, MIT graduate student Barrett Powell thought if they could go one step further: What if we could witness molecular machines in motion?
In a recent article published in Nature Methods, Powell has introduced his invention, named tomoDRGN. This technique is designed to depict the structural variances observed in proteins within cryo-ET data, which stem from protein motions or interactions with different partners- an occurrence known as structural heterogeneity.
In traditional methods, proteins are typically imaged only once in purified samples. However, in cryo-ET, each protein is imaged multiple times from different angles, sometimes over 40 times. This presented a challenge for tomoDRGN due to the overwhelming amount of data. To overcome this problem, Powell ‘upgraded’ the cryoDRGN model to prioritize the highest-quality data.
“By excluding some of the lower-quality data, the results were actually better than using all of the data – and the computational performance was substantially faster,” Powell says.
When imaging the same protein multiple times, radiation damage can occur. As a result, the initial images are often clearer since they suffer less damage, according to Powell.
An interesting result of tomoDRGN came about when the researchers shared raw data showing ribosomes inside cells at near-atomic resolution. Powell applied tomoDRGN to this dataset, unveiling differences in the structure around ribosomal particles. Some of the ribosomes were found near a bacterial cell membrane, where they were participating in a process known as cotranslational translocation. This happens when a protein is being made and moved across a membrane simultaneously.
In addition, tomoDRGN’s demonstrated capability for identifying uncommon structural states within protein populations highlights its accuracy and efficiency. For instance, in another experiment involving the protein apoferritin, tomoDRGN detected a small group of ferritin particles with iron bound to them, making up just 2 percent of the dataset. This discovery emphasizes the method’s ability to spot subtle variations that might be missed by traditional methods.
Powell and his colleagues see many ways to use tomoDRGN to improve our understanding of how cells work. This finding, as they say, has opened the door to generating new theories about how ribosomes collaborate with crucial protein machinery responsible for moving proteins beyond the cell’s borders. They’re especially excited about exploring how it can help with studying ribosomes and other parts of cells.
Algorithms have become indispensable today. You can try this online tool to make text talk like a person!
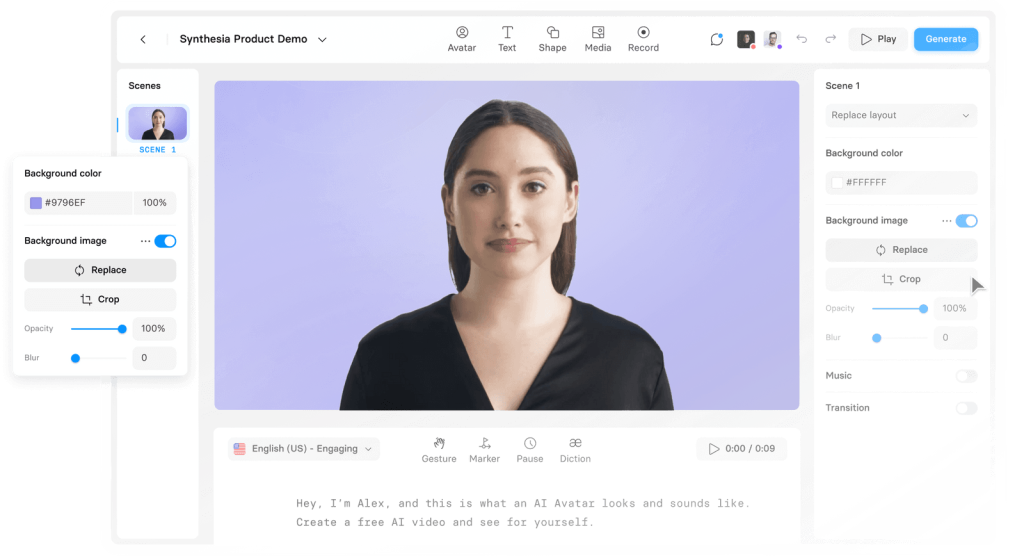
- AI-Powered PCs: Overhyped Trend or Emerging Reality? - August 21, 2024
- Princeton’s AI revolutionizes fusion reactor performance - August 7, 2024
- Large language models could revolutionize finance sector within two years - March 27, 2024