Artificial intelligence reduces a 100,000-equation quantum physics problem to only four equations.
The new approach makes it simpler to find “hidden patterns”.
Physicists must deal with all of the electrons at once rather than one at a time.
A new AI program created by Columbia University researchers has found its own strange version of physics.
The program’s output captured the Hubbard model’s physics even with just four equations.
It took weeks for the machine learning algorithm to train, which required a lot of computational power.
AI has now become advanced enough to handle real-world problems. And it is frequently more effective than humans at doing so, as demonstrated by Alexa, Tesla’s self-driving cars, OpenAI’s GPT3 defeating a human philosopher, and DeepMind’s AlphaZero defeating human chess grandmasters. Adding to this, scientists now claim that by using a method known as neural networks to reduce the mathematical representation used to describe a quantum system, they can learn a lot more about the system.
The new approach also makes it simpler to find hidden patterns, as opposed to just explaining physics and forecasting outcomes for other scientists to find, claim the scientists from Flatiron’s Center for Computational Quantum Physics (CCQ).
Physicists used artificial intelligence to simplify a difficult quantum problem that previously required 100,000 equations into a simple work that only requires four equations — all while maintaining accuracy.
The research may change how scientists examine systems with plenty of interacting electrons. The method may also help in the development of materials with desirable qualities like superconductivity or use in the production of renewable energy if it is transferrable to other issues. Additionally, it may inspire the development of novel technological applications that call for precise models of electrons in many-particle systems, such as quantum computing hardware and software.
“We start with this huge object of all these coupled-together differential equations; then we’re using machine learning to turn it into something so small you can count it on your fingers,” says study lead author Domenico Di Sante, a visiting research fellow at the Flatiron Institute’s Center for Computational Quantum Physics in New York City and an assistant professor at the University of Bologna in Italy.
The significant challenge relates to the electron movement on a lattice that resembles a grid. Interaction occurs when two electrons are present at the same lattice location. Scientists can study how electron behavior leads to desired phases of matter, such as superconductivity, in which electrons flow through a material without resistance, using this configuration, known as the Hubbard model, which idealizes several significant classes of materials. In addition, scientists test the new methods on the model before they use them on quantum systems with greater complexity.
The Hubbard model is quite simple, though. Modern computing techniques and even a small number of electrons are not enough to solve the problem. Because the fates of electrons can become quantum mechanically entangled as they interact, physicists must deal with all of the electrons at once rather than one at a time. This is true even when the electrons are widely separated on distinct lattice sites. The computational difficulty becomes exponentially more complex when there are more entanglements as a result of more electrons.
One method for studying a quantum system is a renormalization group. Renormalization groups (RGs) are formal tools, which scientists use in theoretical physics to systematically assess how a physical system changes when seen from different scales. As the energy scale at which physical processes occur changes, it reflects changes in the underlying force laws (codified in a quantum field theory), with energy/momentum and resolution distance scales essentially combining under the uncertainty principle.
Unfortunately, there may be tens of thousands, hundreds of thousands, or even millions of individual equations that we must solve for a renormalization group that analyzes all potential electron couplings and makes no concessions. The equations are also challenging to comprehend because they each show the interaction of two electrons.
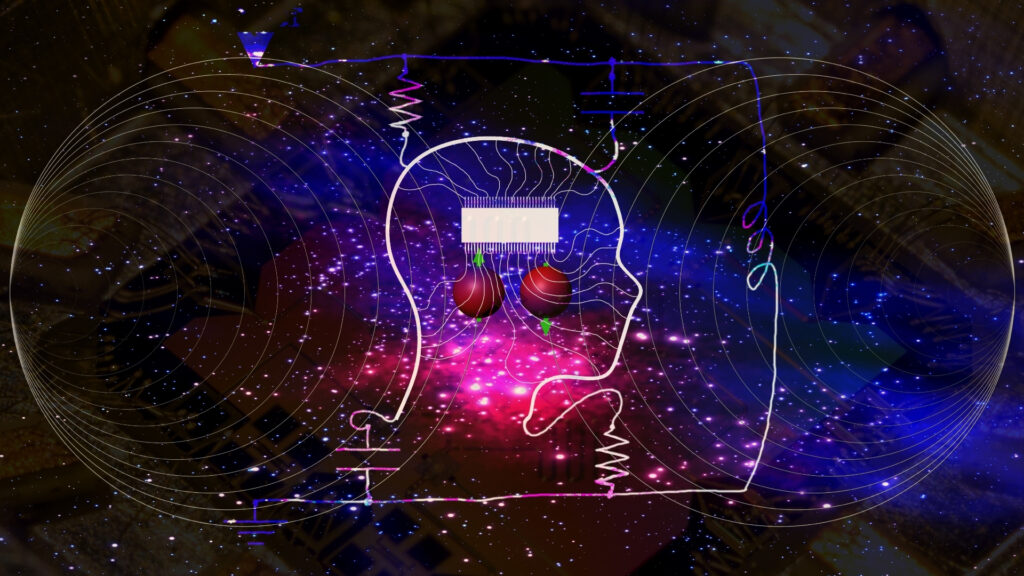
Di Sante and his colleagues questioned whether they could utilize the neural network, a machine learning technology, to simplify the renormalization group. The neural network is like a cross between a frantic switchboard operator and survival-of-the-fittest evolution. First, the machine learning program creates connections within the full-size renormalization group. The neural network then tweaks the strengths of those connections until it finds a small set of equations that generates the same solution as the original, jumbo-sized renormalization group. The program’s output captured the Hubbard model’s physics even with just four equations.
“It’s essentially a machine that has the power to discover hidden patterns,” Di Sante says. “When we saw the result, we said, ‘Wow, this is more than what we expected.’” “We were really able to capture the relevant physics.”
It took weeks for the machine learning algorithm to train, which required a lot of computational power. The good news, according to Di Sante, is that they can modify their curriculum to address other issues without having to start from scratch now that it has been coached. To gain extra insights that could otherwise be challenging for physicists to comprehend, he and his partners are also looking into what machine learning is “learning” about the system.
The main unanswered question is how well the new technique applies to more complicated quantum systems, such as materials with long-range electron interactions. In addition, there are exciting possibilities for using the technique in other fields that deal with renormalization groups, Di Sante says, such as cosmology and neuroscience.
There seems to be a connection between physics and AI. AI recently changed how we perceive physics (see video link above). Yes, you read that right. A new AI program created by Columbia University researchers has found its own strange version of physics.
The AI developed different variables to explain what it saw rather than rediscovering the ones we presently use after being shown films of earthly physical processes.
The progress of our understanding of the universe, quantum physics, and everything else depends on AI experiments like the ones we are currently witnessing. This is so that AI can better understand the most recent statistics by copying and analyzing them. The ultimate goal is to help us understand ourselves better.
As AI advances, there will be plenty more to come. AI has already started, with ‘Physics’ and ‘Art’.
- Artificial intelligence reduces a 100,000-equation quantum physics problem to only four equations - September 27, 2022
- A study suggests watching TV with children may benefit their brain development - September 26, 2022
- Japan’s cyborg cockroach getting ready to assist in disaster relief efforts - September 22, 2022