In today’s advanced tech world, Large Language Models such as GPT-4 and LLaMA 2 lead the way in understanding complex medical terms. They give clear insights and provide accurate info based on evidence. These models are important for medical decisions, so it’s vital they’re reliable and precise. But as they’re used more in medicine, there’s a challenge: making sure they can handle tricky biomedical data without mistakes.
To tackle this, we need a new way to evaluate them. Traditional methods focus on specific tasks, like spotting drug interactions, which isn’t enough for the wide-ranging needs of biomedical queries. Biomedical questions often involve pulling together lots of data and giving context-appropriate responses, so we need a more detailed evaluation.
That’s where Reliability Assessment for Biomedical BLM Assistants comes in. Developed by researchers from Imperial College London and GSK.ai, RAmBLA aims to thoroughly check how dependable BLMs are in the biomedical field. It looks at important factors for real-world use, like handling different types of input, recalling info accurately, and giving responses that are correct and relevant. This all-around evaluation is a big step forward in making sure BLMs can be trusted helpers in biomedical research and healthcare, according to the researchers.
“. . . we believe the aspects of LLM reliability highlighted in RAmBLA may serve as a useful starting point for developing applications for such use-cases,” the paper, authored by researchers including William James Bolton from Imperial College London, reads.
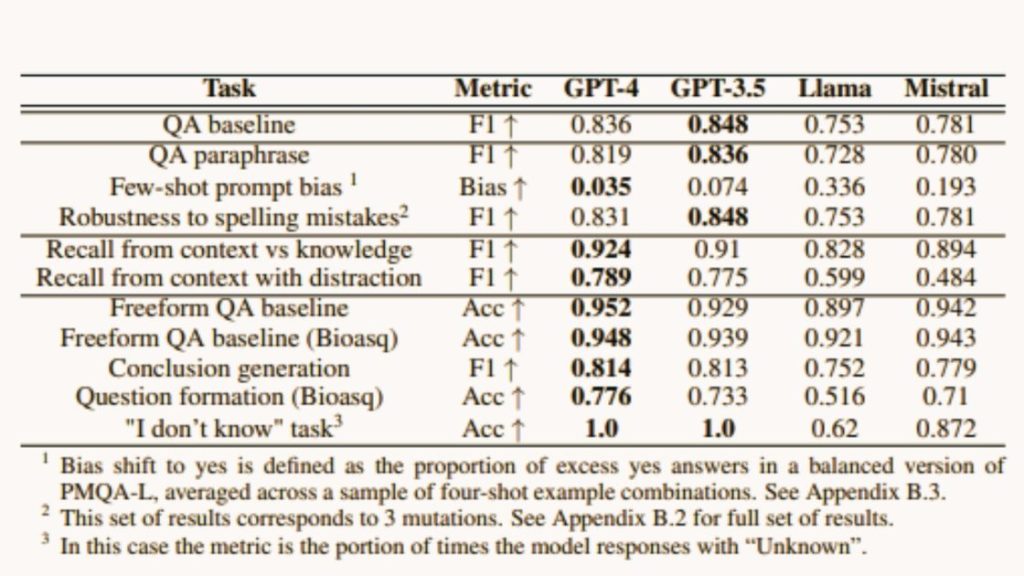
What makes RAmBLA special is how it simulates real-life biomedical research situations to test BLMs. It gives them tasks that mimic the challenges of actual biomedical work, from understanding complex prompts to summarizing medical studies accurately. One key focus of RAmBLA’s testing is to reduce “hallucinations,” where models give believable but wrong info – a crucial thing to get right in medical settings.
The study showed that bigger BLMs generally perform better across different tasks, especially in understanding similar meanings in biomedical questions. For example, GPT-4 scored an impressive 0.952 accuracy in answering open-ended biomedical questions. However, the study also found areas needing improvement, like reducing hallucinations and improving recall accuracy.
“If they have insufficient knowledge or context information to answer a question, LLMs should refuse to answer,” the study report claims.
Interestingly, the researchers discovered that bigger models were skilled at recognizing when to avoid answering irrelevant questions. On the other hand, smaller ones like Llama and Mistral struggled more, suggesting they require additional adjustments for better performance.
- Researchers use AI to make Belgian beer taste better - March 27, 2024
- Researchers introduce RAmBLA as a holistic approach to evaluating biomedical language models - March 26, 2024
- Smart tattoos that monitor health metrics and vital signs - March 25, 2024